The Metrics of Artificial Intelligence: How to “Measure” the Impact of AI in Business
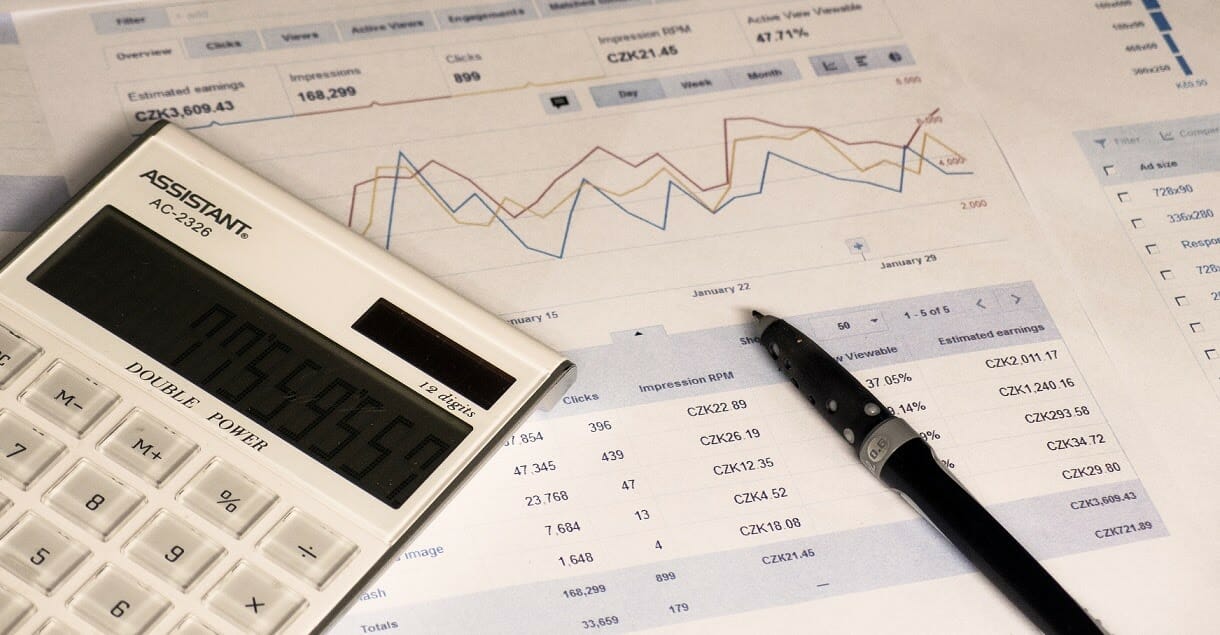
Ok, let’s talk about numbers.
Don’t worry, no math lessons involved.
Instead, we will focus on how to quantify, using proper metrics, the impact of AI on your business.
Numbers and metrics
Each company should know the real status of its processes, activities, and routines.
The right way to know it is to use measurements: metrics.
There are many methods and approaches for quantifying incomes and results, profits and costs, customer satisfaction, eccetera.
But what about AI? How to understand and measure the impact of AI on your business?
If we have to evaluate this impact, we will need some numbers, some metrics.
A practical example
I’ll use an example in the article to make it clearer.
Our example is an abstract “AI Matcher” AI project. The essence of this project is to match two huge databases (several million rows each) with each other and find the best matches.
The owner of the AI Matcher is a hypothetical investment company.
It’s easy to imagine this project in real business: matching goods and customer expectations, matching job offers and candidates, matching signs of illness and possible diagnosis, and so on.
Therefore, this example is quite common for AI projects.
The business metric
Business is money. So … the main business metric is money flow.
Every business task, requirement, goal, and value can be represented as money.
If you have decided to start an AI transformation in your business, an artificial intelligence project should bring you added value.
A good way to understand this is to use measurements. You will need it for at least three good reasons:
1. Project results should be measurable.
At least this will be important for the contract and technical specifications. The project should have clear success and failure criteria.
2. You should know how the project goes.
Is it in the right direction or not? (AI projects can have many right directions). The project development team should know the metric.
A specific feature of AI projects is they can be very unclear for business preliminary results. If you do not have a project metric, you will not be able to understand them and make correct decisions.
3. You should cleverly understand the project value.
This will be extremely important for business planning. One more specific feature of AI projects is that they can be finally tested just on real data in real usage.
So, you need to clearly understand the real impact on your business from AI.
How to specify the metric?
Profit or its money representation will be a very high-level starting point for AI measurement.
How to do it? Simple! Follow these three steps:
1. Choose how to assess the project’s impact on your business.
The impact can be represented as time or resource (materials, money, etc.) savings for employees or manufacturing processes, as an increase in the number of clients, as direct additional profit or savings, and so on.
2. Select the level of impact that you expect to get from the project.
The level should be based on measurements and adjusted for your business.
You can use information about other successful AI projects and you should choose two scenarios for the assessment: pessimistic (low impact level), optimistic (high impact level).
From my experience, the approach with pessimistic and optimistic scenarios is the most accurate and successful, because it is more reliable in case of risk.
For most projects, the difference between these two assessments should be within 20-25%. This limitation will help you to be more careful in your prognosis.
3. Turn your impact levels into cash.
It means you should calculate the profit that the AI project should help you earn.
It will show a real impact on your business, help adjust the project budget, and help make the final decision on project implementation.
An example of a business metric
The project development process is a “moving” entity.
The preliminary results can be confusing. Also, the project may require some adjustments after deployment.
The metric will help you avoid such confusion.
Let me clarify this point with an example.
The metric of an investment firm
The Project Owner, an investment firm, has a department that helps make investments for private investors.
The department has two databases: investor (client) database and investment package database.
The main source of profit is the percentage of profit from a successful investment. A major success factor is the compliance of an investment package with a client’s expectations and capabilities.
Before the AI transformation, all compliance checks were done manually.
Let’s write down some numbers
Rate of successful cases: 90%.
Margin from a successful case: 10 000 EUR.
Loss from an unsuccessful case: 2 000 EUR
The average number of cases: 100 per month.
The goal of the project
Our goal is to maximize the percentage of successful cases and thereby increase profits. We can follow the three-step scheme mentioned earlier for our assessments.
Step 1. The source of the impact is the percentage of successful cases.
Step 2. AI should help increase the percentage up to 96 – 98% (pessimistic and optimistic assessments).
Step 3. Calculations, see Table 1.
According to the table below, the profit from implementing AI Matcher should be between 72000 and 96000 EUR / month. Hence, the value is quite significant.
The project makes sense (from my experience, if the project were real, it would pay off in a month).
Table 1. Business impact assessment
The metric is done. Now, make the right choices.
So, you now have a business impact assessment. This is your metric.
For the AI team, the most important point is the increase in the success rate of 6-8%. You should send these numbers to them.
Right now you are able to follow the development process and its results.
For example, maybe the AI team reaches 95.5% in a shorter time and that will be enough for you. Maybe they will find a way to reach 99% with more time.
Now you can make the right decisions. You have measured the AI in money.
Some bonus information for you
At the end of step 3, the development of the business metrics is finished. You can send the specs to the AI team and wait for the results.
But if you want to go deeper and see how business value turns into technical value, check out the next section. It was written to better understand the development process.
The metric transformation
An AI development team should always operate with corporate values and metrics in their work. But artificial intelligence frameworks and algorithms can’t.
Artificial intelligence is full of beautiful and complicated math. It works with probabilities and accuracy, rates, but also statistical, physical, and mathematical scores.
So, there should be a special technical metric dedicated to AI.
Technical metrics must meet an important requirement: they should be capable of being converted into business metrics and vice versa.
These transformations can have several steps: see the image below.
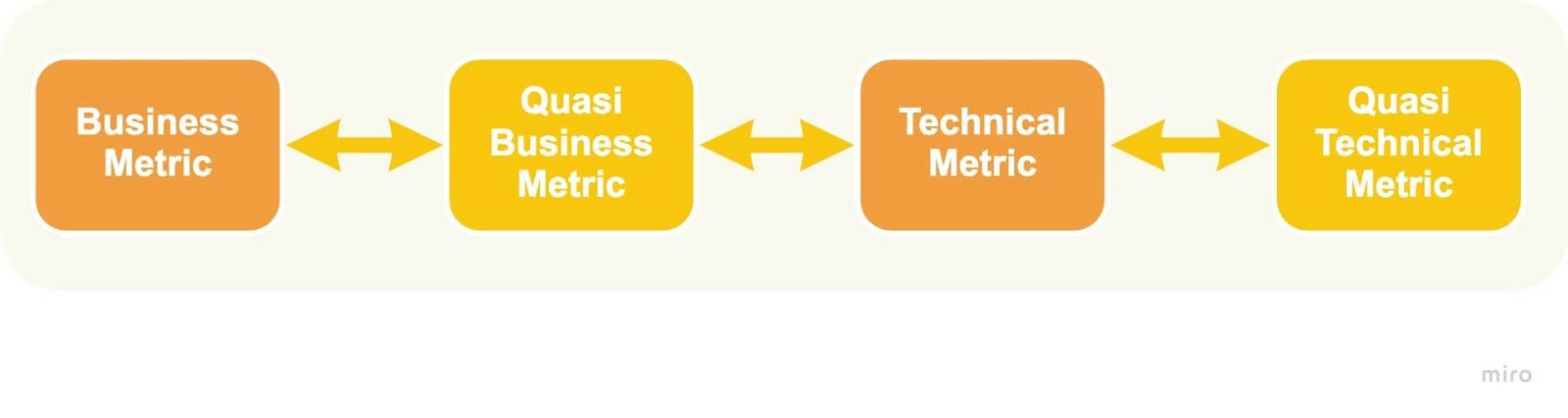
Switching from business to technical metric
The essential blocks are “Business Metric” and “Technical Metric”. But sometimes it is not possible to directly transform business metrics into technical.
For this reason, Quasi Business Metrics should be introduced.
For complicated projects, it can also be difficult to find suitable technical metrics. In this case, the AI team will choose Quasi Technical Metric.
This metric can be customized and developed specifically for your project.
Let me show you!
The transformation applied to our previous case
For the example above, the Business Metric is “Total profit increase” (72 000 – 96 000 EUR / month).
Quasi Business Metric is “Rate of successful cases” (base – 90%, target 96 – 98%).
The rate of successful cases can be easily transformed into a Technical metric. For our project, it can be “accuracy” or “probability of success” (base – 0.90, target 0.96-0.98).
To increase the sensitivity of the AI system up to the correct result, a quasi-technical metric can be introduced (custom metric). This metric will be used directly in the AI training process.
When developing this metric, the AI team can enter rewards for the system in case of correct predictions and penalties for all other cases.
Thus, the numbers for the custom metric can be 0.925 – 0.975 (instead of 0.96-0.98): here can be a large number of options, depending on which path the team takes.
At the same time, the quasi-technical metric can be converted into a business metric.