Supervised Learning, Unsupervised Learning, And More: The Main Types of Machine Learning
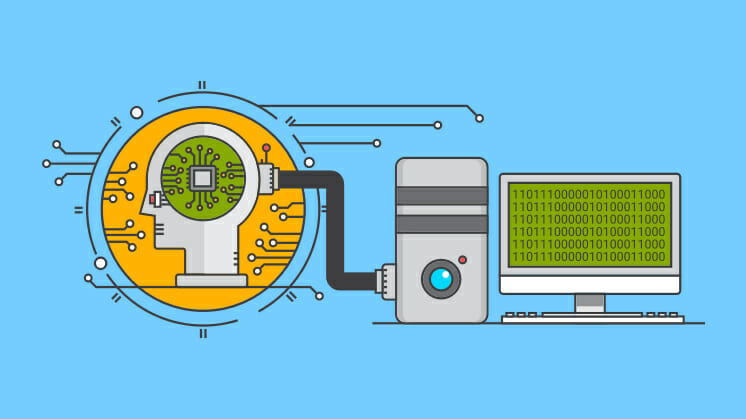
In this article, we’ll focus on the second word contained in the concept of Machine learning, which is… well… LEARNING!
Yep, things are getting serious, so drink a coffee and let’s start.
… Just to avoid tragical misunderstandings, for “coffee” I mean espresso.
Not cappuccino or americano.
Especially after 11 am.
Ok ok, I get to the point. There are four main categories of Machine learning: supervised, unsupervised, semi-supervised, and reinforcement.
Every approach offers different solutions to train our systems. Let’s discover them together!
What is Supervised learning?
Supervised learning is an approach that teaches machines by example.
It involves building algorithms using previously classified examples, having the idea that there is a relationship between the input and the resulting output.
So we are talking about inputs already associated in some way with their outputs.
How does Supervised learning work in practice?
In supervised learning, machines are exposed to large amounts of labeled data.
This means that this data has been annotated with one or many labels.
The process of attaching labels to unstructured data is known as data annotation or data labeling.
Labels can indicate if a photo contains a car or a person (image annotation), what the topic of an essay is (text classification), which words were uttered in an audio recording (audio transcription), and many others.
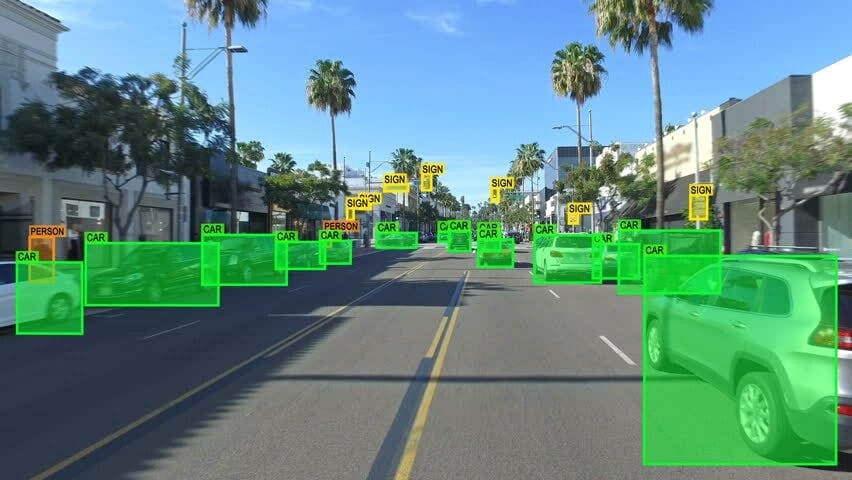
Hello, I see you!
Another interesting example of image annotation?
Images of handwritten numbers annotated to indicate which figure they correspond to.
Given a sufficient amount of examples, a supervised-learning system will learn to recognize and distinguish the shapes of each handwritten number!
Yes, applications that recognize texts from handwritten notes work in this way. Crazy stuff, really.
However, these tools are not powerful enough to recognize my handwriting.
I write SO bad…
Labeling data is really long. Longer than The Divine Comedy!
Training these systems usually requires an incredible amount of labeled data.
Some systems literally need to be exposed to millions of examples, before being able to master a task properly.
That was the case of ImageNet, a famous image database.
Using one billion of photos to train an image-recognition system granted record levels of accuracy (85.4 percent) on ImageNet’s benchmark
The size of training datasets continues to grow. In 2018, Facebook announced it had compiled 3.5 billion images publicly available on Instagram, using hashtags attached to each image as labels.
The laborious process of labeling the datasets is often fulfilled using crowdworking services, such as Amazon Mechanical Turk, which provides access to a large pool of low-cost labor spread around the globe.
ImageNet required over two years by nearly 50,000 people, mainly recruited through this service.
It’s capitalism, baby!
Facebook followed an alternative approach, using immense and publicly available datasets to train systems without the overhead of manual labeling.
“In 2018, Facebook announced it had compiled 3.5 billion images publicly available on Instagram, using hashtags attached to each image as labels.”
Supervised learning problems
Supervised learning problems are categorized into regression or classification. We already discussed them in our introduction to machine learning.
If these concepts are new for you, check it out! Meanwhile, I will give you a fast explanation.
In a regression problem, we try to predict results within a continuous output.
This means that we want to map input variables in a continuous function.
In a classification problem, we are trying instead to predict results in a discrete output.
In other words, we are gonna map input variables into discrete categories or classes.
What is Unsupervised learning?
In contrast, Unsupervised learning is used with data that does not already have a label or classification.
In short, when we don’t already give the “right answer” to the computer. The algorithm will have to independently understand what is provided to it.
We use this system to explore the data and identify any internal structures or patterns.
In short, the machine will identify groups and find relationships or similarities in the data to split them into different categories and groups, known as clusters.
The problem of Clustering
When our goal is to discover groups of similar cases within the data, we talk about clustering.
One example where clustering is used is in Google News, which examines thousands of new stories on the web and groups them into cohesive news clusters.
The news stories that are all about the same topic will be displayed together.
Unsupervised learning is also very common when we want to identify customers with common characteristics to whom we can turn to through specific marketing campaigns.
This is a clear instance of clustering because we have all this customer data, but we don’t know in advance what are the market segments and who is part of each segment.
What we are gonna do is to let the algorithm discover it just from the data.
Practically, unsupervised learning is the favorite approach of lazy people like me.
“One example where clustering is used is in Google News, which examines thousands of new stories on the web and groups them into cohesive news clusters.”
Strengths and weak points of Unsupervised learning
This approach is great for more than one reason:
- There may be cases where we don’t know which classes are the data divided into.
- We may want to use clustering to get a general idea of the data structure before designing a classification method.
- As we have seen above, annotating large datasets is very expensive and therefore we can only label a few examples manually.
On the other hand, unsupervised learning has some flaws:
- It is harder as compared to Supervised Learning tasks.
- With no labels available, we cannot know if our data is significant.
- An external evaluation is strongly recommended.
The Semi-supervised learning approach
In Italy we would say “neither meat nor fish”. Even here in Warsaw, where I live, they say something similar.
But in Polish.
And my Polish is worst than my Italian handwriting. So I don’t understand their jokes.
As you can imagine, Semi-supervised learning mixes supervised and unsupervised approaches.
Let’s see how!
The mechanisms of Semi-supervised learning
The technique is based on using a small amount of labeled data and a large amount of unlabeled data to train our machine.
We talk about pseudo-labeling.
We start by partially training a machine learning model using our labeled data.
Then, that partially trained model is used to label the unlabeled data.
Finally, the model is trained on the resulting mix of the labeled and pseudo-labeled data
The viability of semi-supervised learning has been boosted recently by Generative Adversarial Networks.
We are talking about machine-learning systems that can use labeled data to generate completely new data, for example creating new altered images from existing pictures.
Basically, what we all do during the lunch break with all our stupid apps, but a million times faster and better.
As the effectiveness of semi-supervised learning increases, access to huge amounts of processing power will become more important than access to large, labeled data sets.
What about Reinforcement learning?
We conclude the classification with Learning by reinforcement, very often exploited in video games and for navigation.
The algorithm finds out with which actions the greatest rewards are obtained through trial and error.
Unlike the previous approaches, this type of learning deals with sequential decision problems, in which the action to be taken depends on the current state of the system and determines its future state.
Reinforcement learning and… videogames!
An example of this approach is Deep Q-network by Google DeepMind’s, which was able to beat humans in many vintage video games.
The system is fed with many game match samples and recognizes various information about the game dynamics, such as the distance between objects on the screen or their speed.
It then considers how these dynamics and the actions performed in the game relate to the score achieved by players.
Through the learning process, the system builds a model of which actions will maximize the score in which circumstance.
For example, in the case of the video game Pong, our machine will try to understand how the paddle should move to intercept the ball effectively.
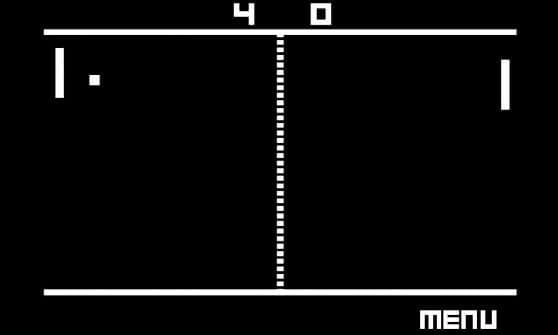
I’m the guy on the left. Yep, I’m a great player.
The three main components of this process are the agent (who learns and makes decisions), the actions (what the agent can do), and the environment (what the agent interacts with).
The agent’s goal is to maximize the reward by reaching the goal in the shortest possible time.
But this approach is not only interesting for video game nerds.
Even the processing of the best car routes can take advantage of this type of approach.
Thanks, Google Maps, you do it damn well. I love you.
“An example of Reinforcement learning is Deep Q-network by Google DeepMind’s, which was able to beat humans in many vintage video games.”
Time to say goodbye… (sung like Bocelli)
Well, our journey through the categories of Machine learning is complete. I hope you enjoyed the trip!
And your coffee.
Espresso.
Remember.