AI, Google Translate, and Linguistics: How Algorithms Help Us Understand Each Other
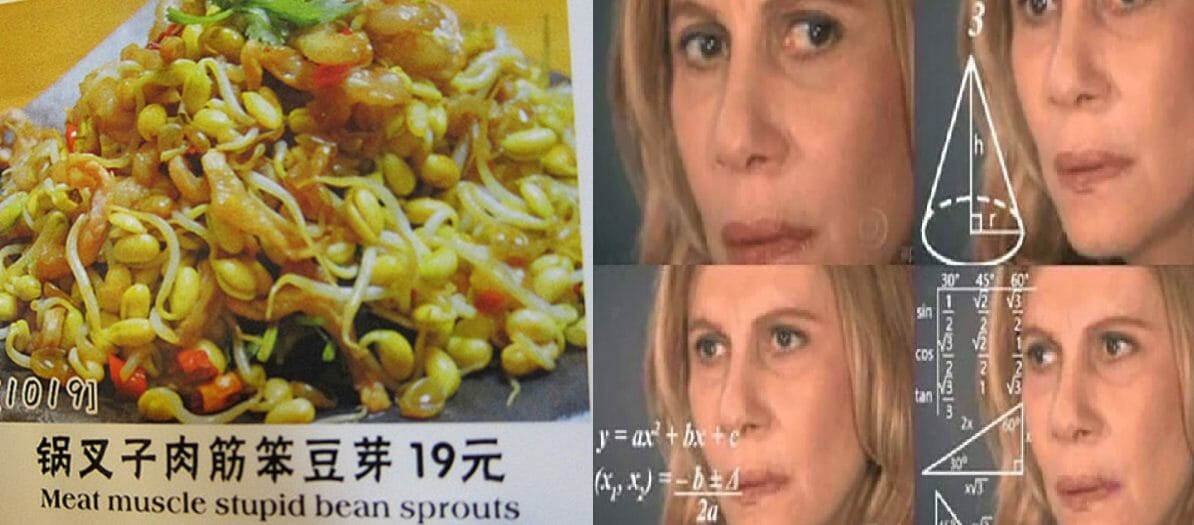
“From sheep to Doggy Style traceability of milk chain in Tuscany.”
Confused? Well, you are not the only one.
This phrase appeared on the website of the Italian Ministry of Education and Research some years ago when online translation tools, forgive my rudeness, literally sucked.
How did we improve from that kind of abomination to Google Translate’s most recent (and pretty impressive) performances?
Short answer? AI and neural networks applied to Linguistics.
Long answer? Read the article!
Google Translate’s Neural network revolution
In September 2016, researchers announced the greatest leap in the history of Google Translate: the development of the Google Neural Machine Translation system (GNMT), based on a powerful artificial neural network with deep learning capabilities.
Its architecture was first tested on more than a hundred languages supported by Google Translate.
The system went live two months later, replacing the old statistical approach used since 2007.
The GNMT represented a massive improvement thanks to the possibility to manage zero-shot translations, in which a language is directly translated into another.
In fact, the former version translated the source language into English and then translated the English text into the target language.
But English, as much as all human languages, is ambiguous and context-related. This could cause funny (or embarrassing) translation errors.
Why is GNMT so damn efficient?
GNMT’s brilliant performances could be achieved by considering the broader context of a text to deduce the most fitting translation.
How? GNMT applies an example-based machine translation method (EBMT), in which the system learns over time from millions of examples to create more natural translations.
That’s the essence of deep learning!
The result is then rearranged, considering the grammatical rules of the human language.
This involves the GNMT’s capability of translating whole sentences at a time, rather than just piece by piece. In addition, it can manage interlingual translations by recognizing the semantics of sentences, instead of memorizing phrase-to-phrase translations.
After this brief overview, let me show you in detail how the first statistical approach worked and how neural networks made it obsolete.
How did Statistical Machine Translation work?
Before the implementation of neural networks, statistical machine translation (SMT) was the most successful machine translation method.
It is an approach in which translations are processed considering statistical models whose parameters are derived from the analysis of bilingual text corpora (large and structured sets of texts).
A document is translated according to the probability that a string in the target language is the translation of a string in the source language.
To do so, the system searches for patterns in millions of documents and decide which words to choose and how to arrange them in the target language
The first statistical translation models were word-based but the introduction of phrase-based models represented a big step forward, followed more recently by the incorporation of syntax or quasi-syntactic structures.
How to find the best corpora for Google Translate?
Google got the necessary amount of linguistic data from the United Nations and European Parliament documents, which are usually published in all six official UN languages and so represent a great set of 6-language corpora.
Bravo Google, getting something useful from the United Nations seemed impossible but you did it!
Statistical translation pros
“Old chicken makes good soup”. Is that actually true?
Well, partially yes. The old statistical approach had some tricks up its sleeve.
First, it represented a significant improvement over the even older rule-based approach, which required an expensive manual development of linguistic rules and didn’t generalize to other languages.
Even Franz Josef Och, Google Translate’s original creator, questioned the effectiveness of rule-based algorithms in favor of statistical approaches.
In fact, SMT systems aren’t built around specific language pairings and they are definitely more efficient in using data and human resources
Secondly, there is a wide availability of parallel corpora in machine-readable formats to analyze. Practically a giant menu to choose from.
The SMT cons
On the other hand, statistical translation can have a hard time managing pairs of languages with significant differences, especially in word order.
That’s because of the limited availability of training corpora among pairings of extra-European languages.
Other issues are the cost of creating text corpora and the general difficulty of this approach in predicting and fixing specific mistakes.
Fun Fact of the day: even with all these flaws, Google still uses statistical translation for some languages in which the neural network system has not yet been implemented.
A touch of vintage, I guess.
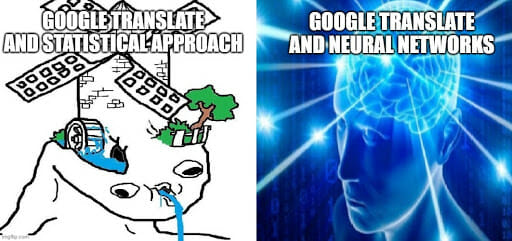
Google’s Neural Machine Translation system
Ok, time to talk about the new neural network-based system, our “enfant prodige”, as the French would say.
(I didn’t use Google Translate, I speak French or something that sounds like French).
As we said, Google has used artificial neural networks since 2016 to predict the likelihood of a sequence of words and to process entire sentences in a single integrated model, while statistical approaches used separately engineered subcomponents.
The old structure consisting of a translation model and a reordering model is therefore abandoned in favor of a single sequence model that predicts one word at a time.
This new system requires just a fraction of the memory needed by SMT models and implies that all parts of the neural translation model are trained together (end-to-end) to maximize its performances, unlike the older ones.
I will not delve into the features of artificial neural networks now. Check out my previous article to refresh your memory!
The neural network’s mechanism
Initially, the word sequence processing was carried on by using recurrent neural networks (RNN).
A first bidirectional recurrent neural network (the encoder) took care of encoding a source sentence for a second RNN (the decoder), which predicted words in the target language.
The main issue concerned the encoding of long inputs, which could be compensated by an attention mechanism: practically, the decoder focused on different components of the input while generating each word of the output to better understand the context.
Later, Convolutional Neural Networks or “Convnets” were introduced granting better results, especially when assisted by attention mechanisms.
Google Translate isn’t perfect (wow, really!?)
Google Translate likes gentlemen. It’s a fact.
If you write a well-structured formal text with short and clear sentences, the cyber God of translations will be pleased and reward you with a good output.
Things change when you talk like One Punch Mickey O’Neil from “The Snatch”.
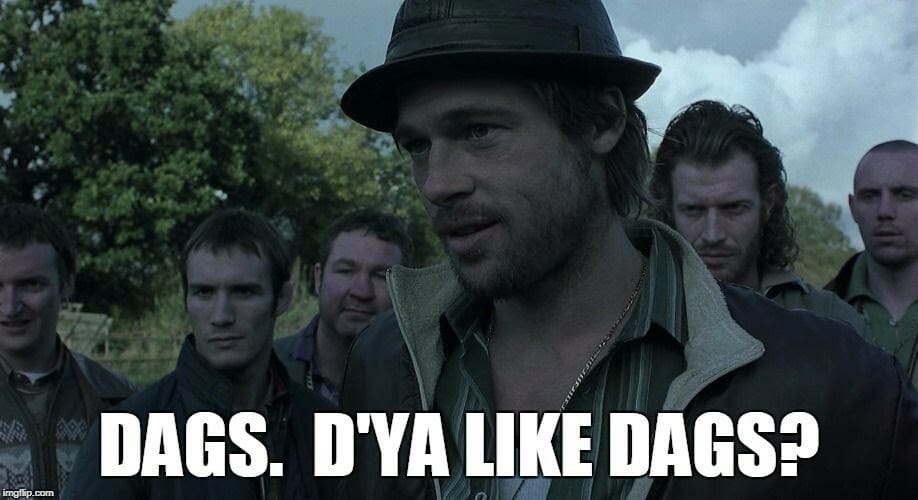
Generally, the system struggles to work properly with long phrases, informal texts, and slang forms.
It is also particularly inaccurate in translations of single words due to their frequent polysemic nature (among the top 100 English words, each has on average 15 different senses) and the lack of context usually given by a full text.
In that case, Google will try to guess taking into account statistical factors, based on what it learned from its immense dataset.
Google Translate’s grammatical weaknesses
Other weak points are related to grammar.
For example, Google Translate struggles to recognize between the perfect and imperfect aspects of the Romance languages or to understand the subjunctive form.
It also confuses quite easily between the second person singular or plural.
The frequency of these mistakes may vary greatly among languages, due to different levels of research, investment, and availability of digital resources to train the system.
According to research conducted through human evaluations, the main idea of a text translated from English into another language is understandable more than 50% of the time for 35 languages. In the other 67 languages, the threshold of 50% is not reached.
AI still needs us
Google Translate is an amazing tool driven by artificial intelligence.
BUT…
Believe it or not, one of the best ways to improve its performance is still human intervention.
In this regard, a crowdsource application was released by Google in 2016 to offer translation tasks and collect human feedback.
Users can translate phrases, agree/disagree on a given translation, or edit a Google’s translation if they think they can improve the result.
Over four years, the “suggest an edit” feature granted an improvement in 40% of cases.
That’s the proof, fellow humans, that we are still cool!