The 4 Types of Data Analytics to Enhance your Business
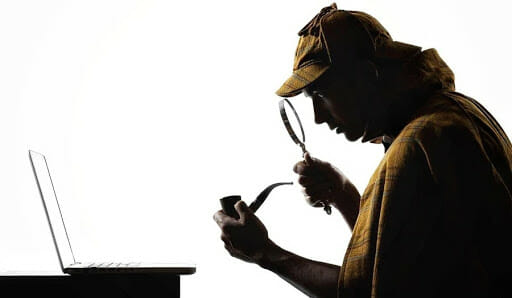
According to Sherlock Holmes: “It is a capital mistake to theorize before one has data. Insensibly one begins to twist facts to suit theories, instead of theories to suit facts.”
Thanks, Sherlock! We took your words so seriously that we came up with 4 different types of data analysis:
- Descriptive analytics
- Diagnostic analytics
- Predictive analytics
- Prescriptive analytics
Which data analytics do you need?
Data analytics is an essential tool to optimize the overall performance of any company. By implementing some type of data analytics in their business models, companies will be likely able to make better decisions, optimize their processes, and reduce costs.
But what is the best type of data analytics for your business model?
Let’s find out starting from the definition of its four main categories, which we will list in order of complexity and, at the same time, of potential impact and benefits.
1. Descriptive analytics
This type of data analysis describes what happened in a given time. It digs through data from multiple sources to provide precious insights into the past.
It could be about the number of views on your videos or the sales volume of a particular product on your e-commerce site.
This is a relatively simple but essential approach to deciding, for example, which product categories to invest in and how to better manage reordering and inventory.
The downside is that descriptive analytics highlights a phenomenon without explaining in detail why it happened. That’s why such an approach should always be combined with other types of analytics to give your business real benefits.
Simple, but useful!
Despite being the simplest form of analysis, descriptive analysis is critical because it deals with converting large data sets into a small amount of information that is easier to understand.
It typically does this by summarizing and highlighting the main features that interest us, also using graphs and other user-friendly representations.
2. Diagnostic analytics
The next step is to put together the historical data and give an explanation of what happened. Diagnostic analytics focuses precisely on the reasons for a certain event or trend.
Is the increase in orders the consequence of your latest marketing campaign? Has the African heatwave doubled ice cream sales?
Data is more important than ever!
The key to the success of this approach is the wide availability of data. Like descriptive analytics, diagnostical analytics require “internal” past data.
But, unlike the previous one, diagnostic analytics often includes external information from a wide range of sources to determine in detail what happened.
You may realize, for example, that your website revenue has decreased in the last quarter. This can be linked to a decrease in advertising spending but also a change in Google’s algorithm.
To find clues to this trend, it will be necessary to isolate data from different sources such as conversion rate, new registrations, and so on until a suspicious pattern is found.
3. Predictive analytics
Predictive analytics provides you with clairvoyance superpowers. It helps companies understand what is likely to happen in the near future by leveraging the data previously collected with descriptive and diagnostic analysis.
Once clusters and patterns in raw data are detected, it is possible to build models that will forecast future trends and events.
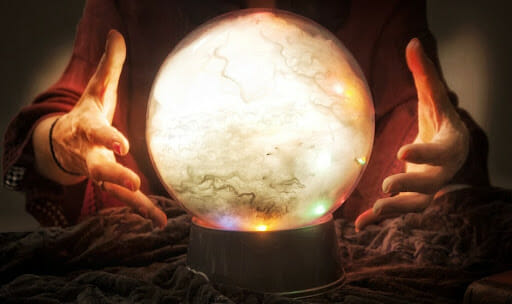
Pros and cons
Such an approach is particularly advanced and brings many advantages, for example in the energy field where companies need to know in advance future fluctuations in production and consumption to optimize distribution through the power grid.
It is also a rather common tool in the arsenal of marketers, particularly in the context of sentiment analysis.
An algorithm can be trained to recognize positive and negative customer comments based on sample texts to autonomously predict the tone of future comments and give insights into overall sentiment.
At the same time, this type of analysis is heavily based on very expensive technologies such as machine learning (ML) and deep learning (DL) and requires a massive availability of high-quality data to function properly.
4. Prescriptive analytics
The purpose of prescriptive analytics, which is certainly the most advanced category on our list, is to suggest a course of action to avoid future problems or to get the maximum benefit from a promising trend.
This type of analysis is widely used, for example, by recommendation engines to determine buying patterns among similar clients and provide them with personalized recommendations based on their browsing activity.
To this end, recommendation systems analyze customer data collected through descriptive analytics (such as previously purchased products) and predictive analytics (e.g. market segmentation) to recommend the most suitable product for a specific customer.
Recommendations should always be tested and validated against the desired result, for example by verifying that consumers have actually clicked on the recommended product or service. Such a feedback mechanism helps improve performance over time.
ML for prescriptive analysis
Prescriptive analytics, as much as predictive analytics, works best when powered by machine learning and deep learning algorithms, with all the pros and cons that come with it and that we’ve already mentioned above.
It is a particularly data-hungry approach and requires both internal and external data sources to be exploited to its full potential.
For this reason, any company that wants to implement prescriptive analysis in its business processes should carefully evaluate the possible costs and compare them to the expected benefits.
The most popular types of data analytics
According to the “2016 Global Data and Analytics Survey”, descriptive analytics was the type on which company executives relied most for their decision making (58%) in the category “Rarely data-driven decision- making”.
However, diagnostic analytics dominated with 34% in the “Rather data-driven” category, while predictive analytics was ranked first in the “highly data-driven” category with 36%.
What does it mean? Well, basically these results tell us that any choice regarding the type of data analytics depends to a large extent on the development phase of a company.
The more a company relies on informed decisions, the more it will be necessary to implement the most advanced types of analysis.
The growing trend of advanced analytics
In the last years, we have seen a shift of attention towards the more advanced types of data analysis, namely predictive and prescriptive analytics.
This trend seems confirmed by more recent surveys. For example, a majority of the “2018 Advanced and Predictive Analytics Market Research” respondents indicated for the first time advanced analytics as “critical” or “very important”.
A majority of the “2018 Advanced and Predictive Analytics Market Research” respondents indicated for the first time advanced analytics as “critical” or “very important”.
Can we afford advanced analytics?
On the other hand, we shouldn’t forget that more advanced data analytics also means increased costs, requirements, and risks.
Some useful insights in this regard come from the 2020 BARC’s study “The Future of Analytics”, which is based on a global survey of more than three hundred professionals working with advanced analytics.
66% of respondents declared to lack the human resources necessary to carry out advanced analytics.
According to the study, in fact, “using advanced analytics across the entire company only works when data access and governance is right and when enough users possess the relevant skills”.
The right data analytics type for your business
As you may have already realized, the different types of data analysis are not mutually exclusive but, rather, they coexist and complement each other.
But what’s the right mix for your business? To understand this, you should take into account your current progress in implementing data analytics and your actual need to dig deeper into the data in order to get better insights and more effective decision making.
Generally, the best way is to gradually move from the simpler and cheaper types of analysis to the more complex, expensive, and challenging to implement.
Just remember that every extra step you take comes at a cost and risks giving disappointing results in terms of ROI. So… choose wisely and don’t overdo it!