AI and Machine Learning in the Wind Power Industry
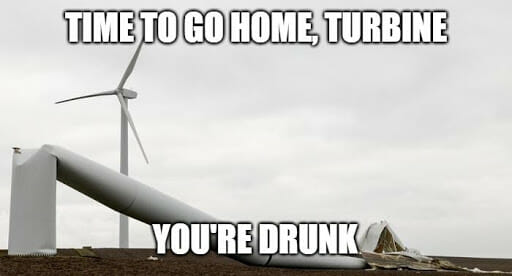
If you ended up here, it’s probably because you are HUGE FANS of wind turbines.
Eh eh eh … my humor sucks.
Okay, techy guys! In today’s article, we’re gonna focus on the contribution of AI and machine learning (ML) to the wind power industry.
Specifically, you’ll find out how algorithms can help us:
- increase the accuracy of weather forecasts to predict the performance of wind farms (yep, no wind = no energy)
- support plant monitoring and maintenance tasks
- make electricity grids smarter
Wind energy nowadays
In an effort to avoid a toxic Blade Runner-style environment, countries are seeking to diversify their energy sources and rely more on cleaner ones.
Speaking of wind, this source can be exploited through turbines, which are positioned onshore (on land) or offshore (at sea).
Today, the majority of wind farms are built offshore to enjoy stronger and more stable wind conditions.
Besides, larger units can be transported and deployed easily, also causing less visual disturbances and potential conflicts of interest.
What about wind energy issues?
Is everything so cool? Well, not exactly.
Firstly, the maintenance costs to ensure optimum performance over their 20-25 year lifespan are quite high, especially in offshore locations. They can represent up to 25% of the offshore installation.
Secondly, wind energy production is very unstable, due to a wide range of external factors, starting with weather conditions.
On windless days, grid operators have to resort to conventional power plants to meet demand, buying energy on the spot market to supply the grid with prices above the average price.
Energy producers can also be penalized with fines from governments for power outages.
OUCH!
Too much wind energy can be even worse
On the other hand, when weather conditions allow 90% of the daily needs to be covered by wind farms, energy operators must rapidly reduce the supply from gas and coal plants to avoid overloading the entire network.
In addition to the risk of overload, producers are not compensated for the extra energy in case of exceeding the expected supply thresholds. And no one likes to work for free.
The economic weight of this variability is far from negligible. In Germany alone, the costs of adapting wind energy inputs to the power grid amount to approximately $ 550 million annually.
“In Germany alone, the costs of adapting wind energy inputs to the power grid amount to approximately $ 550 million annually.”
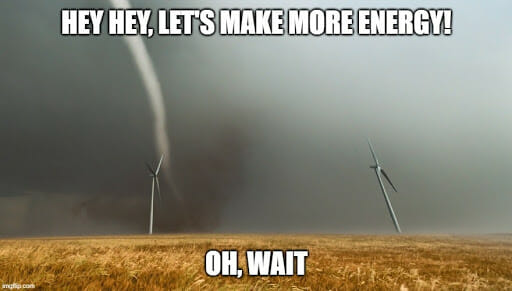
How AI can help the wind energy industry
In search of a solution to these problems, the major players in the energy market have turned to AI-based tools in order to maximize the strengths and reduce the flaws of wind power generation.
And when I talk about artificial intelligence, I’m mostly referring to machine learning and deep learning.
ML is a subpart of AI. It focuses on creating computer algorithms that can automatically learn from data and improve their performance through experience.
ML algorithms can identify structures and patterns in data sets, build mathematical models to represent these relations, and use them to make predictions or decisions without being explicitly programmed.
The latest evolution of machine learning is known as deep learning (DP). This recent branch of AI mimics the mechanisms of the human brain to process information by using deep artificial neural networks (ANNs).
1. AI and forecasting
After all these nerdy technicalities, I guess you want to know how ML algorithms can help us tackle the issues mentioned above, right?
A good example to understand it is the initiative of DeepMind and Google, which have implemented ML to produce about 700 megawatts of wind power in the central United States.
The system developed by DeepMind, an English company purchased by Google in 2014, uses machine learning algorithms and neural networks trained on past weather forecasts and historical data on turbines.
It can predict wind power production 36 hours before the actual generation, allowing operators to schedule optimal hourly input to the power grid one day in advance.
Much better than the weather forecast in Belarus. NEVER TRUST THEM!
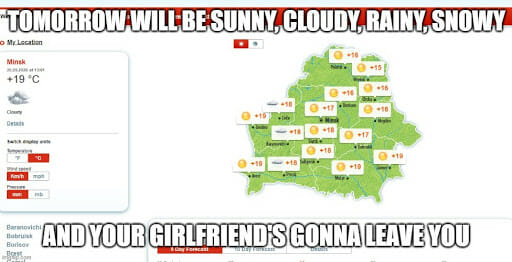
Knowledge is power, knowledge in advance is a superpower
An efficient forecasting system is an advantage that should not be underestimated. That’s because programmable energy sources are more valuable on the market, as they can provide a certain amount of electricity at a specific time.
Translated into economic terms, accurate forecasting of the next day’s production is essential for successful financial market operations.
In fact, the value of wind energy produced with this system has grown by about 20 percent.
Yep! In the end, it’s (also) a matter of money. Welcome to reality!
Not only Google
The project of DeepMind and Google is just the tip of the iceberg.
Nowadays, machine learning algorithms are widely applied in the wind energy industry to enhance forecasting activities and stabilize solar energy production.
The most common are probably SVMs (support vector machines) and gradient boosting decision trees, but experts are testing many different models and discussing which ones are most efficient in different locations and weather conditions.
What is certain, is that every machine learning-based model will work better if it has been trained with data collected on a specific site and during the time of year for which predictions need to be made.
This will likely increase predictive performance over using a trained generic model with a representative dataset of many weather regimes.
Basically, these models aren’t so much multitasking. Maybe someday researchers will create female ML models and everything will be easier.
2. AI for Operation and Management
Another interesting application of AI in the field of wind energy is that relating to the operation and management (O&M) of systems.
Thanks to artificial intelligence and in particular to machine learning algorithms, it is possible to accurately evaluate the potential downtime of components.
This leads to an increase in plant productivity, a reduction in component replacement costs, and a longer overall life expectancy.
Machine learning for Condition monitoring
An efficient way to reduce O&M costs is to predict failures before they occur, using a robust condition monitoring (CM) approach.
Condition monitoring involves observing the components of a wind turbine to detect changes in its operation that could be a sign of future failure.
Recent developments in sensors and signal processing systems, combined with machine learning algorithms, offer new opportunities for efficient and rapid CM analysis.
CM examines the various activities of the plant, detecting faults in the blades, the temperature of the generator, the power curve…
This data is collected by specific sensors and processed through a wide range of ML-based methodologies. In particular, neural networks (NN), support vector machines (SVM), and decision trees are the most commonly used.
What’s the best ML model for monitoring?
Selecting the best machine learning model is like choosing a dessert in a bakery. Terribly difficult and questionable.
The choice basically depends on the problem to be solved, because each one can be more adequate in the management of specific data sets and activities.
In general, however, we can say that deep NNs, capable of learning complex nonlinear functions, can achieve better performance than more traditional systems
3. AI and smart grids
According to AMR (Allied Market Research), the smart grid market is set to grow from $ 67 billion in 2017 to $ 170 billion by 2025. A business that is therefore not only efficient for energy sector players but also very profitable.
Almost as profitable as hand sanitizer gels in 2020.
But how can grids be “smart”?
Well, we are talking about automated power grids that can monitor and control every consumer and node. This ensures a continuous flow of information on the status of the system.
Monitoring is based on a dense network of smart meters assigned to each user, as well as special devices known as synchrophasors, which measure voltage and current in various points of the network.
These sensors allow the grid to change the flow of electricity as needed, lowering the workload and lowering energy prices.
Aware of the potential of this technology, the US Department of Energy has already invested over $ 4.5 billion in smart grids.
The private sector is implementing such systems too, including Google for its data centers.
“The US Department of Energy has already invested over $ 4.5 billion in smart grids.”
What can go wrong with smart grids?
The flip side of this potentially fantastic system is the risk of disastrous cyber attacks.
In fact, a centralized data collection system is an easy target for hackers interested in destabilizing the network and stealing valuable consumer information.
To cope with similar situations, some British researchers are developing protection systems based on blockchain technology.
Yes, the same as Bitcoin: a decentralized ledger system that would allow us to avoid the massive storage of user data in a single point.
That way, my bill details will be safe too and no one will ever know that I’m a damn squanderer.
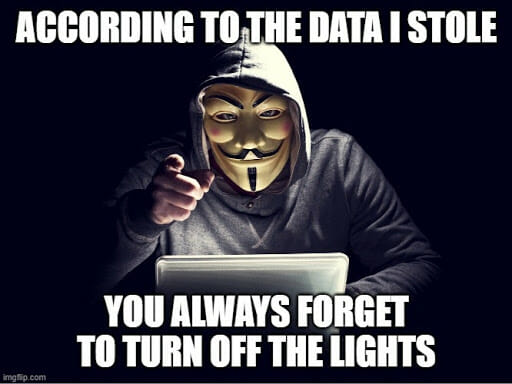
Wind energy is cool and makes good money
Artificial intelligence, when applied in the field of renewable energy, is able to do something often considered impossible: to combine public and private interests, generating advantages for both.
It represents an essential tool for maximizing the production of clean energy and preserving the environment.
At the same time, it boosts the performance and competitiveness of market players, making this business more attractive.
In the near future, this dualism will help foster research and likely ensure the rapid implementation of artificial intelligence technologies.
As fast… as the wind!