The Unexpected Love Affair Between Tinder and Artificial Intelligence
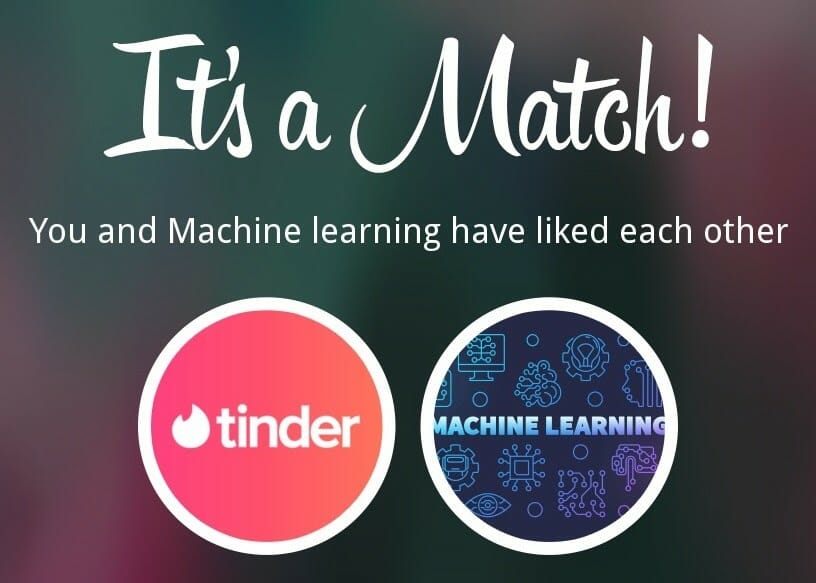
I’m REALLY happy to talk about this subject for at least two good reasons.
Firstly, AI and machine learning applied to Tinder is definitely a spicy conversation topic.
Believe me, it will be a fascinating journey to discover the artificial intelligence algorithms that make Tinder so damn effective.
Secondly, telling my wife that I’m inquiring about Tinder in the name of science is priceless!
AI is everywhere, also in Tinder!
Unfortunately, the development team behind Tinder isn’t particularly inclined to reveal the secrets of its algorithms. So the technical information available to us is quite limited.
Anyway, it’s still enough to get a general idea of how AI, and more specifically machine learning, are used by this app.
After a little research, I found three main fields of application:
Recommendation system
Tinder implements a machine learning-based algorithm to generate personalized recommendations.
Harassment checking
The app uses ML to automatically screen for potentially offensive messages.
“Smart Photos” feature
Machine learning helps users identify which profile pics are the most successful.
Before delving into these topics, let’s introduce the two protagonists of our research: Tinder and machine learning. It shouldn’t be a surprise for you. It was all written in the title.
What is Tinder?
Tinder is an online dating application to find the great love of your life, get married, and have children have fun.
“Fun”.
The mechanism is pretty straightforward. Users create a personal profile with pics, interests, and a small bio.
Then, they can check other profiles and swipe to like or dislike them. Once two users have “matched” with a mutual like, they can text each other.
Considering that every sentient being in the universe already knows about Tinder, we can probably move on.
What about Machine learning?
If you came here for that “machine learning” in the intro, probably you already know what we are talking about. So, I’ll make it short.
Machine learning (ML) is a branch of artificial intelligence. It focuses on the creation of computer algorithms which can improve their performance automatically through experience.
ML algorithms are able to recognize specific patterns in sets of data, build mathematical models to represent them, and use these models to make predictions or decisions without being explicitly programmed.
A Polish colleague once told me that ML is essentially magic. If this Harry Potter-style explanation is not enough for you, take a look at my two articles about machine learning and its main categories.
I wrote them with the same love with which my grandma cooked Italian tortellini.
“ML algorithms are able to recognize specific patterns in sets of data, build mathematical models to represent them, and use these models to make predictions or decisions without being explicitly programmed.”
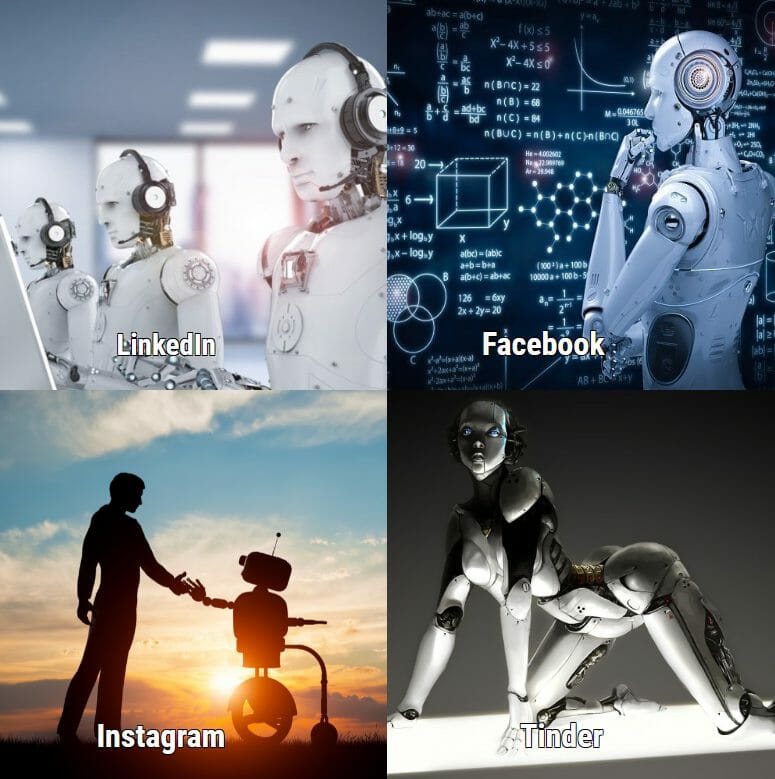
Tinder and ML: the Recommendation system
After the previous theoretical premise, it’s time to analyze the ways in which AI and ML contribute to Tinder’s mysterious functioning.
Firstly, Tinder uses VecTec, a machine-learning algorithm paired with artificial intelligence to generate personalized recommendations.
According to Tinder chief scientist Steve Liu, Tinder users are defined as Swipes and Swipers.
Each swipe made is mapped on an embedded vector that represents the possible characteristics of the user. For example, hobbies, education, interests, professional career…
When the system recognizes a similarity between two built-in vectors (which means that users share common traits) it will recommend them to each other.
This process allows Tinder’s algorithms to progressively improve their performance in identifying users who could co-swipe and match.
Do we speak the same language? It’s a match!
An additional ML-based algorithm that contributes to successful matchings is Word2Vec.
Let’s say that this algorithm is Tinder’s personal linguist.
In fact, while TinVec analyses the users’ characteristics and learns from large amounts of co-swipes, Word2Vec focuses on words. Specifically, it identifies the communication style of users (slangs, dialects, choice of words based on the context…).
Taking into account this data, similar swipes are grouped together in clusters and the users’ preferences are represented through the embedded vectors that we already mentioned.
Users with similar preference vectors will be mutually recommended by the system more often.
Pros and cons
As we have seen, Tinder learns the kind of profiles you like and dislike based on your previous swipes left or right, in combination with these profiles’ characteristics.
This allows the app to customize its recommendations for you.
On the other hand, we should be aware of some unpleasant implications when we rely on algorithms to make a selection of people based on their attributes.
The risk is to promote a system of classification and clustering which tends to keep away less desirable profiles from the “alpha” ones.
An example? Back in 2014, the famous dating website OkCupid published a study about racial bias in users’ preferences and behavior.
Based on that research, the discrimination of black women and Asian men seems to be pretty common in online dating environments.
Pretty much what happens to nerdy guys every day. But that’s another story.
Machine learning vs Harassment
Tinder relies on machine learning to automatically scan potentially offensive messages and evaluate if some user is a bit too much flirty or just a maniac.
When the system detects a controversial message, Tinder asks the receiving user a confirmation of the potential offense and direct him/her to its report form.
As you can imagine, this mechanism can falter in many borderline situations.
First of all, sensibility towards a certain type of communication changes radically from person to person.
Second, a flirty language perceived as vulgar in many situations can be perfectly tolerated or even appreciated in a dating context.
The algorithm knows if you are a bad boy… or a bad girl.
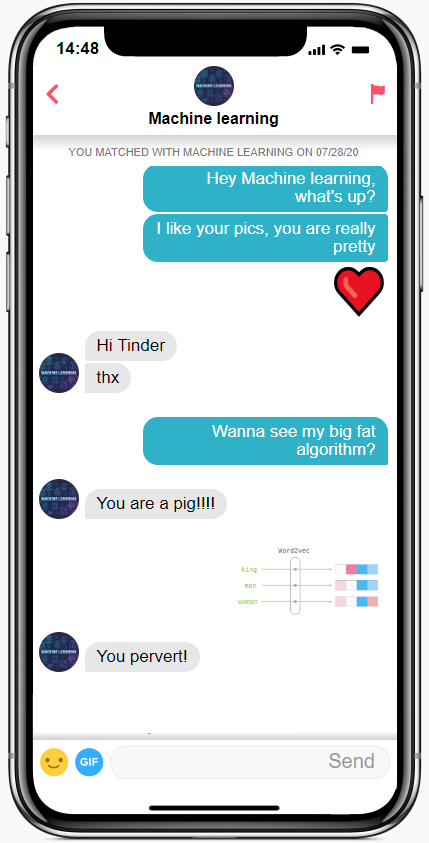
How can an algorithm understand if some shameless Casanova crosses the line?
Tinder’s developers tried to solve this problem by training its machine-learning model on a wide set of messages already reported as inappropriate.
In this way, the algorithm recognized keywords and patterns that could help it recognize offensive texts.
However, Tinder has struggled to mark potentially harmful messages because words can mean different things in different contexts.
Theoretically, the algorithm can still improve in predicting which texts are inappropriate and which ones are not, as it is exposed to more and more data.
For example, the ML-based system progressively learns which messages are generally harmless from a repeated “no” to the user confirmation request mentioned above.
Tinder’ Smart Photo feature
Smart Photo is like the guy in our office who always suggests to his colleagues which pics to upload on their Tinder profiles.
That guy is me. Probably cause I come from Milan, the capital of fashion. So I’m expected to be a great esthete.
Anyway, this feature maximizes your chances of getting a match by identifying which photos work and which don’t.
Guess what? It is based on Epsilon Greedy, a machine learning algorithm!
The mechanism is pretty straightforward. It randomly swaps the first pic seen by other users when your profile is shown on Tinder.
Based on their responses (right or left swipe), it reorders the photos to show your “best” one first.
The company declared on its blog that this feature improved the chances of matching by 12%.
Still not as effective as me. Modestly.
“The company declared on its blog that Smart Photo improved the chances of matching by 12%.”
The algorithm adapts to its users.
The algorithm can also adapt to users’ personal preferences, taking into account their swiping patterns when choosing which photo to show first.
And thanks to its ML-based nature, it gets more and more efficient with each input. This means that the more you swipe and the more you’re swiped on, the better the algorithm works.
Let’s assume that your most successful photo shows your satisfied face as you bite into a giant rare steak.
Well, in case your profile is viewed by a vegan, Tinder will choose something less bloody as the first image.
For example, a pic of your satisfied face as you bite into a giant… Uhm… stone???
Is Tinder’s algorithm the new Cupid?
Machine learning, like many other technological innovations connected to AI, is showing tremendous effects on the vast field of human relations.
Perhaps online dating is one of the most frivolous aspects of its influence.
Frivolous, but still fascinating and remarkable.
As we have seen, ML-based algorithms help maintain a safe online environment and connect users most efficiently.
Should we say that Tinder’s algorithms are some sort of Cupid’s modern version?
Well, maybe. But I still have some doubts about it.
At least, algorithms don’t have those weird heart-shaped arrows.
And that creepy childish expression.