Machine Learning Guide for Newbies
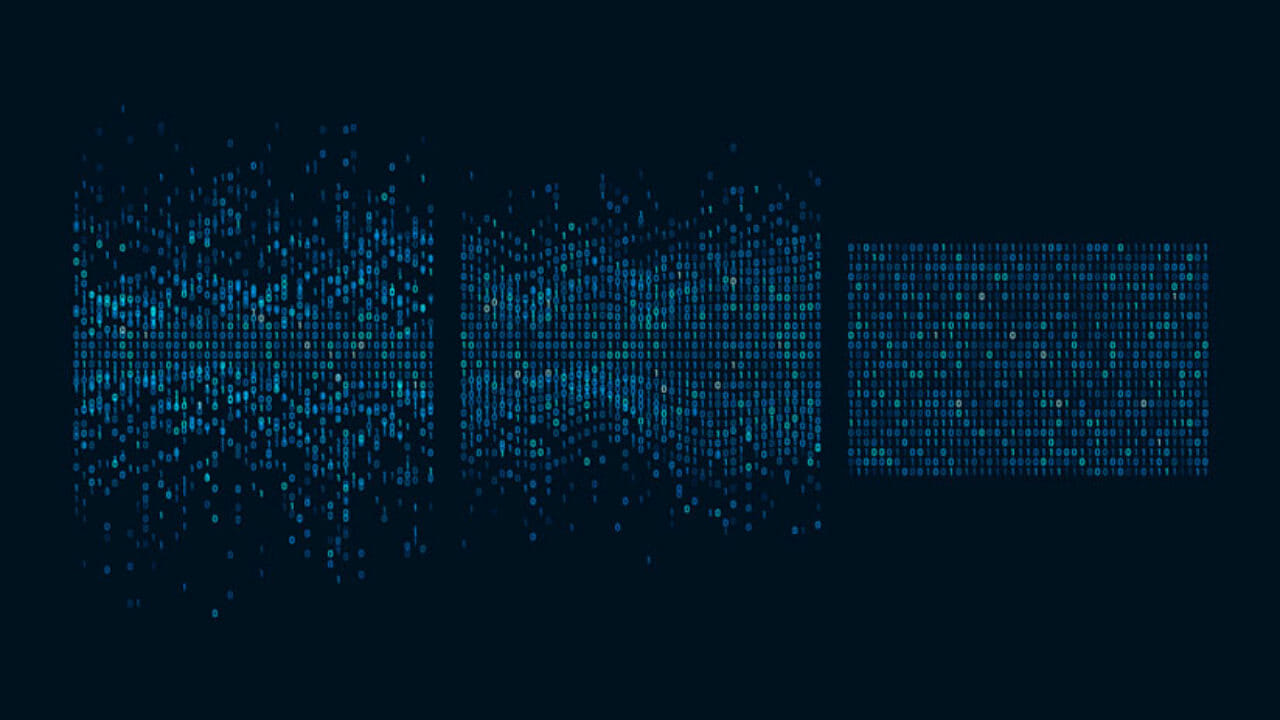
Concepts like machine learning and artificial intelligence sound weird and mysterious?
No worries!
I will accompany you to discover this fascinating world starting from the basics.
So, finally, the technological secrets that permeate our modern daily life will be clearer to you.
Machine learning surrounds us!
You wake up, take some bread and heat it in the microwave, which in a few seconds does its job as expected.
You drive to work and your smartphone recommends you to choose another way because of an accident. Even today you have avoided a dozen insults.
Are you Italian like me? More than a dozen.
After four hours in the office, you spend your lunch break biting a sandwich and scrolling photos on Tinder, who seems to know you better than your best friend.
How do you say? You have never used Tinder?
Of course, my dear, of course. Me neither! BLINK BLINK.
After the break, you spend your time PRODUCTIVELY on the laptop browsing Netflix, which recommends several interesting new titles.
Magic? No, Machine learning!
All these interactions between you and the machines now seem obvious and automatic.
Yet, if you think about it, they have something almost magical. This magic, which is NOT magic, is called machine learning.
Excluding the microwave.
That really works by magic.
What is Machine learning?
Machine learning is basically a branch of artificial intelligence.
More specifically, it explores the study and construction of algorithms that can learn from a set of data and make predictions about them.
In other words, our algorithms will inductively build a model based on samples. It is, therefore, a process closely related to pattern recognition.
In the field of computer science, machine learning is a variant of traditional programming.
It provides systems the ability to automatically learn and improve from experience without being explicitly programmed.
This translates materially into having algorithms that can give interesting information about a set of data, without having to write any specific code.
Instead of writing the code, the data is entered into a generic algorithm and the algorithm generates its own logic based on the data entered.
“Machine learning provides systems the ability to automatically learn and improve from experience without being explicitly programmed.”
A mathematical example to understand Machine learning
To give a small example, traditional programming provides an X input and an f function that processes it giving, as a result, an Y output.
In machine learning we could instead give the computer a series of inputs and the related outputs, asking it to find out which mathematical function connects them.
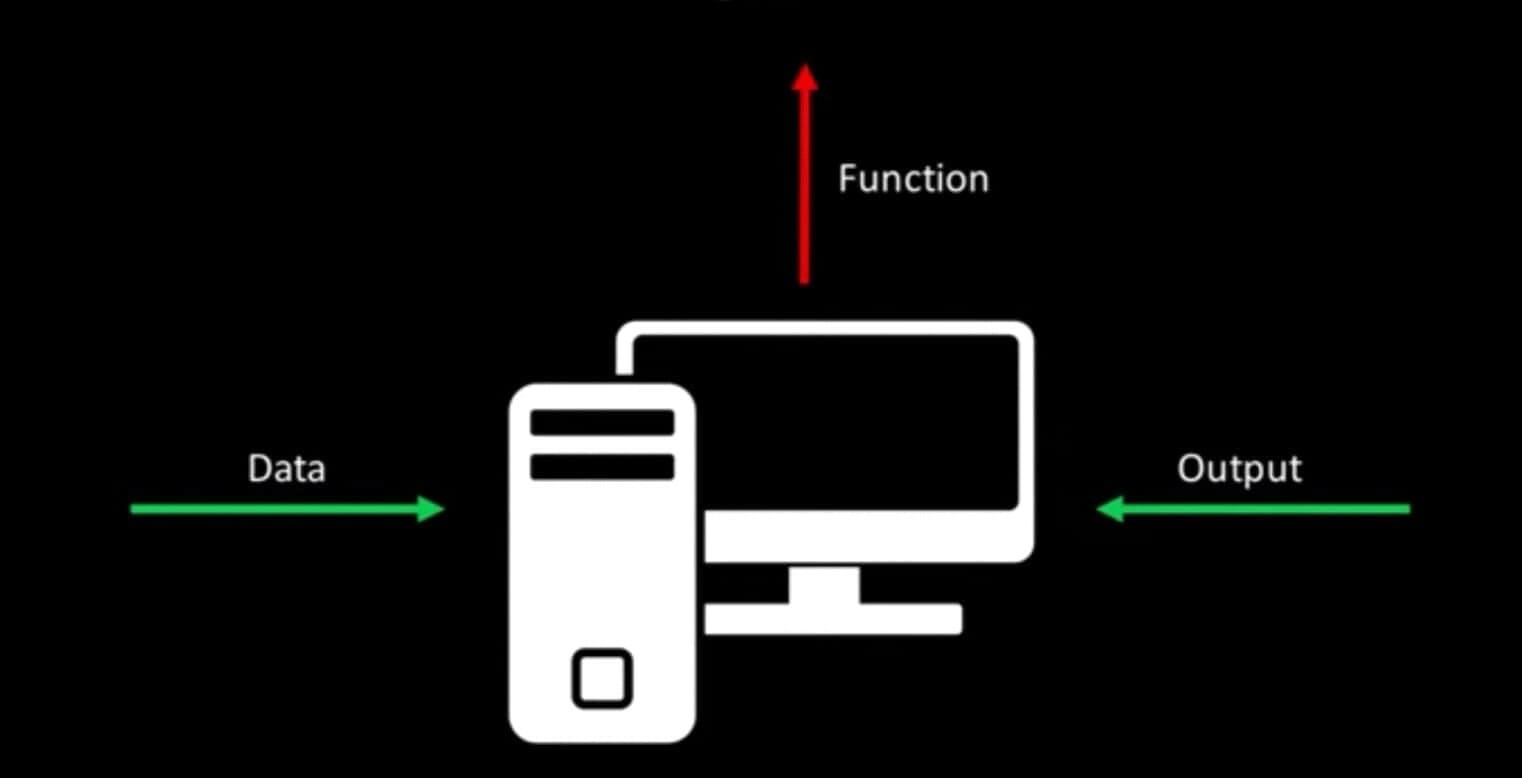
We talk in this case of supervised learning and we will analyze the concept in detail later.
NO SPOILER!
Traditional programming: Y = f (X)
Machine learning Y = f (X)
We could trivially provide the machine with the numbers you see in the table below.
It will identify the correct function, which in this case is obviously the squared elevation.
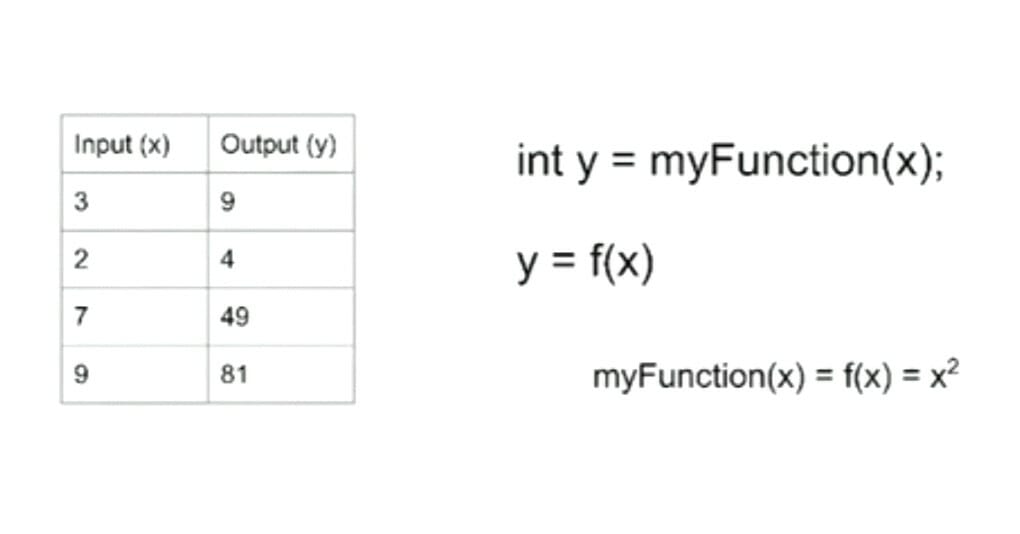
Machine learning… in practice!
You don’t like numbers? Do you hate math?
No fear!
Let’s move on to a more practical example, very common among experts in the sector to explain the concept of machine learning to beginners.
It is the legendary house example. Let’s imagine we are a real estate company.
Over the years, we have accumulated hundreds or thousands of data relating to real estate sales.
At this point, our problem is to find a link between the square footage of houses and their potential commercial value.
We decide to do it through machine learning.
In essence, the computer will have to identify the connection between input (square footage) and output (price).
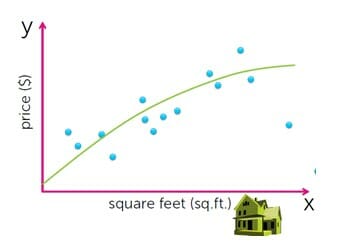
Exactly like our agents are already able to do it through their intuition developed after years of experience.
How does Machine learning represent reality?
At this point, it is better to stop for a second and give a couple of definitions.
The values of the houses that I will have to predict are called labels. The values I enter as input are the features.
Finally, every sample I give to the computer is a data point.
Okay, I gave three definitions and not two.
Be patient.
Once the input and output data have been entered, the machine will try to process a function that connects them through the generic algorithm mentioned above.
This is a process that goes through numerous attempts and errors, in which we try to obtain a model that deviates as little as possible from reality.
In short, we aspire to obtain the smallest possible gap between the model’s expectations and the actual results.
What is the Cost function? Reality vs expectations
This difference between model and reality is calculated through the so-called cost function.
It calculates what in the image below is represented graphically as the distance between the dots and the curve of the model.
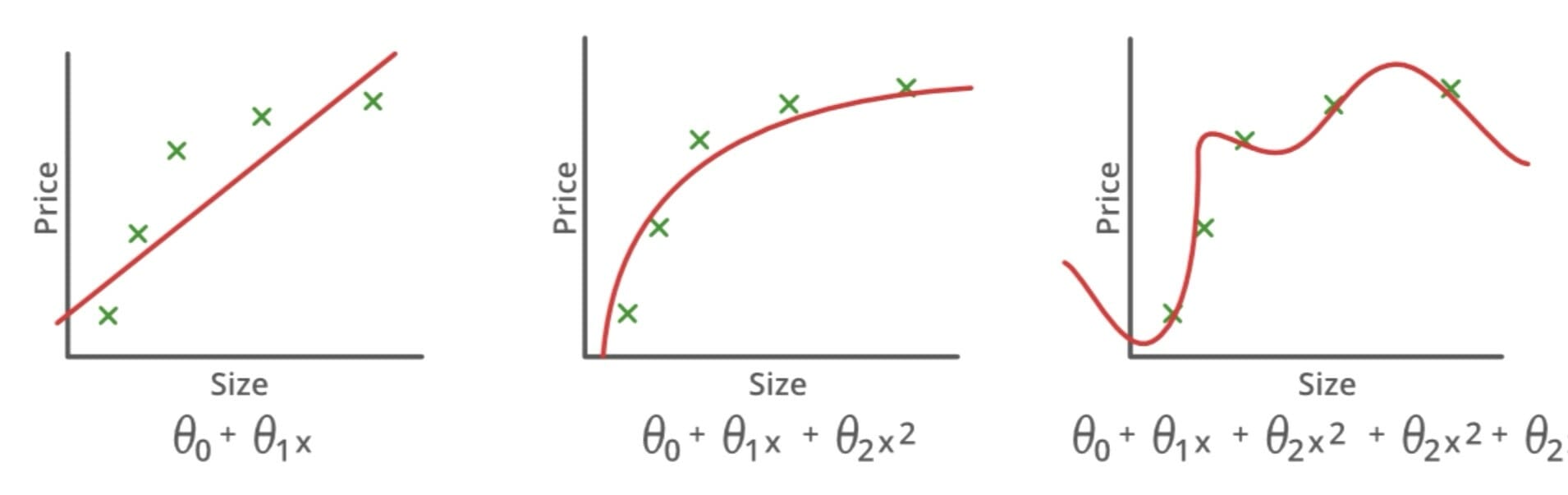
However, the truth is that, as you can see in the image, a model cannot be perfect. The first example is clearly inappropriate, i.e. underfit.
The second, on the other hand, already seems to us to be more consistent with the data.
Finally, the third is the one with the lowest cost. Practically the curve coincides perfectly with all the dots.
What is missing from the latter model is generalization!
How do we improve our models in Machine learning?
Generalization is the ability to adapt adequately to any new data without fossilizing only on the initial ones.
Otherwise, the model will tend to overfit, as in the third example, and will, therefore, be perfect for the data of the first test but not representative in a different context.
The solution to this problem is to divide the data between the two parts, namely the training set, and the test set.
The algorithm will then be evaluated based on its performance with data it has never seen, practically on its ability to generalize.
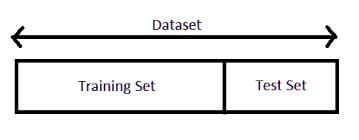
Obviously, the example of the house is extremely simplified because the value of a home will depend on many other factors including the area of the city, the distance from public transport, the view, etc.
This means that the models applied in reality must be extremely more complex.
This amount of work is managed by neural networks, which we will discuss in the next articles.
Maybe.
If you behave well.
Two main problems of Machine learning: Regression vs Classification
Now I am interested in offering you another relatively simple example to clarify the main two problems we may face.
The first is called regression and we have just analyzed it with the case of the real estate agency.
We speak of regression when we have inputs and outputs with continuous numerical values, for example with the probability of success, the value of an asset, the age of the subject in a photograph …
The problem of Classification
The second category is called classification. In this case, we will determine belonging to a class.
For example, we might want to know if there is a dog or a cat in a photo.
Let’s move on to a classification case. You all know Spotify, right?
Come on, this is not Tinder, you don’t have to be ashamed!
Our problem is to understand if a song might please a user. The “like” or “don’t like” results are our labels.
What are the features instead? Well, it depends a lot on the subject, but we could consider the duration, the pitch of the voice, the gender of the singer, the speed, some keywords, etc.
We have here a so-called content-based recommendation problem.
Basically, we have to recommend new content to users based on the characteristics of that content.
The “trick” of Community based recommendation
A similar situation also exists in Pinterest, where photos similar to those already liked are recommended.
Or in Netflix, which offers users new movies to watch.
In all these cases, a very performing system is the community-based recommendation.
In practice, I ask the user community to rate the content they enjoy.
Then we cross the data with a matrix factorization technique:
John likes songs A, B, and C on Spotify.
Mike likes songs A, B, and D on Spotify.
I will, therefore, recommend song D to John and song C to Mike.
Thanks to a similar system, Amazon has increased its performance by about 35%. Not bad at all, right?
“Thanks to Community based recommendation, Amazon has increased its performance by about 35%.”
Some interesting fields of application for Machine learning
Machine learning, as we have seen in the previous examples, is truly omnipresent in our daily life.
Still not convinced? Untrusting!
Here are some more…
Financial services:
banks use machine learning to identify investment opportunities, or to identify customers with risky profiles.
To this, we can add IT surveillance and the detection of potential fraud.
Energy sector:
to find new energy sources by analyzing the soil, to prevent breakdowns in refinery sensors and to streamline distribution processes.
Healthcare:
to analyze patients’ health in real-time through sensors. In this way, doctors are assisted in identifying alarm signals and in developing diagnoses.
Transport:
fundamental in identifying patterns or trends and developing the most efficient routes.
Machine learning is, therefore, a valid tool both for public transport and for delivery companies.
Marketing:
used on websites to propose interesting articles to specific targets, for example by analyzing the history of their purchases.
The use of data to personalize the shopping experience or marketing campaigns can certainly be considered the future of e-commerce.
Public administration:
it is used to sift information useful for public security or for better management of services to citizens, with a consequent saving of money.
It can also be used against identity theft and fraud in general.
How do we train our machines?
For now, everything should be clear enough, right? So, let’s take a few minutes to talk about the different ways to train our system!
The main approaches to learning are supervised, unsupervised, and reinforcement.
What is Supervised learning?
Supervised learning involves building algorithms using already classified examples.
So we are talking about inputs already associated with their outputs.
It is commonly used in situations where historical data allow us to predict possible future events.
For example, it helps us predict whether transactions with some credit cards can be fraudulent, or which customers in a company could seek compensation.
If you have been careful, you will certainly have already understood that the example of the house fell into this category.
Right? Good boy/girl!
What is Unsupervised learning?
Unsupervised learning is used instead with data that does not already have a classification.
The algorithm will have to independently understand what is provided to it.
We use this system to explore the data and identify any internal structures or patterns.
In short, we identify groups and find relationships or similarities in the data.
It is very common in the processing of transnational data, for example when we want to identify customers with common characteristics to whom we can turn to through specific marketing campaigns.
It is also useful for discovering the main differences between various consumer segments.
Finally, it can be applied to notice anomalous values with respect to trends.
What about Learning by reinforcement?
The last case is Learning by reinforcement, especially used in video games and for navigation.
The algorithm finds out with which actions the greatest rewards are obtained through trial and error.
The three main components of this process are the agent (who learns and makes decisions), the actions (what the agent can do), and the environment (what the agent interacts with).
The agent’s goal is to maximize the reward by reaching the goal in the shortest possible time.
Don’t forget the importance of data!
Speaking of learning, we cannot forget a fundamental but often overshadowed aspect, namely the dataset.
The “menu” of data made available to the machine is of crucial importance as it deeply determines the quality of learning and, consequently, the final result.
The best qualities of data
Our dataset should be:
Large:
it should, therefore, present a large number of cases, which may even be thousands or millions depending on the problem to be managed.
Returning to the example of the photo of the cat (yep, I like kittens), we will propose thousands of photos of cats to the computer. The same amount that you could find in a group chat of your mother with her 50-year-old friends.
Various:
there will be cats of all breeds, fur colors, positions, sizes, etc. Yes, even those hairless alien cats, known as sphynx.
Balanced:
the sample must have a fairly homogeneous number of different variables inside. So the computer will analyze a similar amount of photos of cats and … dogs? Tigers? I leave it up to you.
A fairly common trick to greatly expand our dataset without too much effort is the augmenting.
Basically, we artificially generate many new images by applying distortions to the originals (for example by changing light, applying blur …).
“The menu of data made available to the machine is of crucial importance as it deeply determines the quality of learning and, consequently, the final result.”
Conclusions and coffee break
KNOCK KNOCK.
Are you still awake?
Not me.
After the first half of the article, my hand started to move by itself on the keyboard. So let’s put on paper some conclusions and go to relax.
Machine learning is a fundamental aspect not only of programming itself but of our life.
It solves problems otherwise impossible to manage, opens many new perspectives, and is here to stay long.
But above all, it ruined my illusions about a world full of magic.
I’m gonna eat a lot of chocolate to compensate for this trauma.