Big Algorithm is Watching You: How AI impacts on Video Surveillance
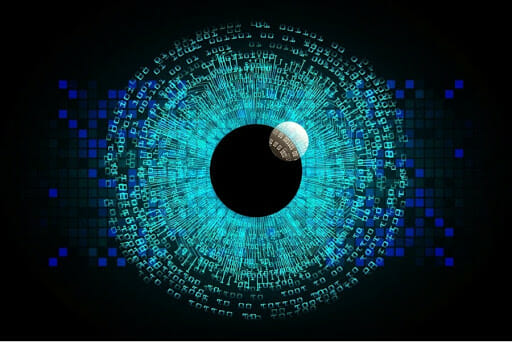
Do you feel observed?
Well, probably cause you are.
*Anxiety intensifies*
Wherever you come from, some form of AI-based video surveillance has probably already been implemented in your country.
In today’s article, we will discover how this technology works and why we need it so much.
Ready Go!
What is AI video surveillance?
AI-based video surveillance relies on computer software to analyze the images and audio of video surveillance cameras. The goal is to identify human beings, objects, or specific situations.
This kind of software is generally developed to define restricted areas within the camera’s view and send an alert when it detects something that should not be there at that moment.
For example, an unauthorized vehicle in a parking lot, an intruder in a private property, or your colleague who is trying to steal your sandwich exactly NOW.
If you don’t believe me, turn around and see for yourself.
Why do we need AI?
The human ability to monitor live surveillance footage has its limits. A guard can keep an eye on a single video monitor for something like twenty minutes before losing 95% of his attention span.
After that, our poor security officer will no longer have a sufficient level of attention to recognize significant events.
These limitations led to the demand for AI tools to better serve the task.
Personally, I face the same issues with my students, but I usually opt for a very loud voice and choreographic hand gestures rather than artificial intelligence.
Italian style.
The rule-based approach for AI surveillance
Video surveillance tasks can be managed by AI using machine vision, a series of algorithms (basically mathematical procedures), which compare the object detected with thousands of recorded samples.
If the AI notices some common traits between this object and the stored images, and these similar features exceed a certain percentage, the system sends an alert.
Many factors can be taken into account: size, width, movement speed, and so on.
This approach is known as rules-based because it involves a set of rules coded by human programmers that cause a warning if violated.
Nowadays, rule-based AI surveillance is the most common type.
The problem of active environments
Rule-based video analytics is cheap and reliable for the majority of security applications, and that’s why it’s still the most common technology.
But there are some specific situations in which it cannot work properly. For example, active environments.
Active environments are locations with high human activity all the time, such as university campuses and hospitals.
In these cases, it’s extremely hard to set rules which can effectively discriminate between simple frequenters and criminals.
The next approach we’ll talk about can solve these issues pretty well!
Behavioral analysis and machine learning
In recent years, AI video surveillance has started to follow a different approach based on behavioral analysis, thanks to the implementation of machine learning technologies.
In this case, the software is fully self-learning and doesn’t require initial programming input.
The AI autonomously understands the typical behavior of humans and objects based on the analysis of patterns of various features (speed, size, orientation, color, shape…).
To do so, it classifies and tags the things and patterns it sees, creating and perfecting its own definitions of what is “normal” and what instead represents an anomaly.
Basically, AI learns to recognize when things “break” the pattern though experience.
For example, the system will understand that a car moving on a road is absolutely normal, but the same car driving on the pavement is not.
That’s okay just if you are playing Carmageddon.
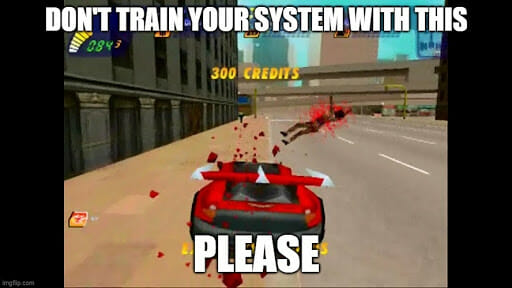
Behavioral analysis in practice
How can this approach help solve the issue of active environments?
Ok, imagine a camera installed on a controlled access door. Every day, the surveillance system sees workers passing through this entrance one at a time by holding their personal proximity card close to a sensor.
This repeated pattern will start to be considered by the system as a standard situation.
At that point, if a person opens the door as usual and a second one grabs the door and sneaks in, our AI will think that something is wrong and send an alert.
Deep learning in video surveillance
The advent of deep learning represented a formidable leap in the evolution of AI technologies.
Deep learning is a subgenre of machine learning that mimics the mechanisms of the human brain to process information.
Artificial neural networks, which are the heart of deep learning systems, have made possible the abrupt improvement of computers in handling complex tasks.
Deep learning-based algorithms are superior to other solutions because they allow us to train and enhance systems with better and wider datasets.
Thanks to this feature, they can reach 99.9% accuracy for certain tasks, while traditional AI algorithms usually cannot achieve greater than 95% accuracy.
Talking about video surveillance, we can find several applications that take advantage of deep learning.
Deep learning for face recognition
According to tests done by the National Institute of Standards and Technology (NIST), facial recognition error rates have dropped by three orders of magnitude over the past 20 years.
Why? Thanks to deep learning, of course!
Today, most facial recognition products rely on deep learning tech.
As shown by researchers from Tel Avis University, their accuracy reached 99.9% for recognition systems in controlled environments, such as airport immigration applications.
That 0.1% is probably Cher after yet another plastic surgery.
Deep learning for person detection
Deep learning is also the main responsible for the recent improvements in person detection.
The moving areas that contain a person are identified through a series of computationally efficient image processing steps.
Later, the areas are processed by convolutional neural network classifiers.
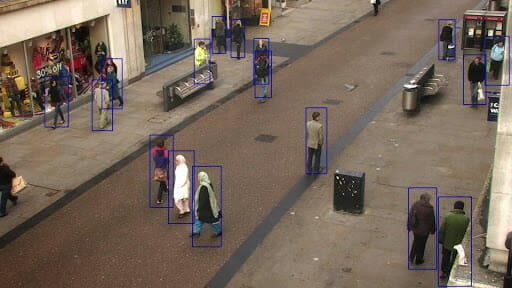
A pair of issues
Deep learning systems are as cool as a Ferrari. And as expensive as TWO Ferraris.
Ok, let’s say MANY Ferraris.
It’s definitely not cheap tech. It requires wide resources in terms of data to train the systems and computational power to fuel them.
Another problem is that not all video analytics algorithms are best applied with deep learning. For example, license plate recognition tools work very well with older computer vision-based algorithms.
AI-based video surveillance around the world
According to the Carnegie Endowment for International Peace, the use of AI for public surveillance is spreading faster and to a wider range of countries than expected.
75 out of 176 countries globally already use artificial intelligence applied to surveillance tasks, including facial recognition systems (64 countries) and smart police (52 countries).
The undisputed leader of AI video surveillance is China.
Absolutely not expected, uh?
The land of cute pandas, majestic dragons, and concentration camps massively counts on these technologies in its cities but is also a major exporter of AI-based surveillance systems.
Chinese companies provide their technologies to over sixty countries, offering soft loans that entice even relatively poor states to buy from them.
Only Huawei offers its products in at least 50 different countries.
“75 out of 176 countries globally already use artificial intelligence applied to surveillance tasks”
AI surveillance in the Free World
Liberal democracies are also key actors in developing and implementing AI surveillance technologies.
In fact, 51% of them deploy AI systems such as facial recognition, automated border controls, and algorithms to predict crimes, against a mere 37% of closed autocratic states.
US companies, for example, are very active in world exports and supply 32 countries with their surveillance technologies. The list of American leading exporters includes IBM, Cisco, and… Palantir.
Lord of the Rings quotes spotted!
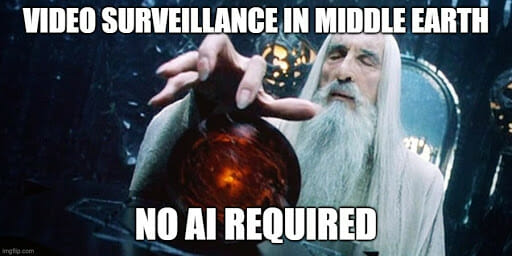
A danger for democracy?
Does it mean that democracies are more paranoic than dictatorships regarding their citizens?
Well, no. Firstly, liberal democracies USUALLY perform better economically and thus can afford such expensive technologies.
Secondly, using AI for video surveillance in these countries doesn’t mean that they will abuse these systems. These technologies can play an important role in supporting authorities to fight terrorism and many other threats.
As the report claims, whether or not governments use it for repressive purposes depends on the quality of their governance.
Balancing security and freedom
However, modern democracies are facing the problem of finding a balance between security and civil liberties, and crossing the line is something that has happened more than once.
For example, in 2016, the Baltimore police secretly deployed drones for public surveillance of residents. The drones were equipped with facial recognition cameras to detect and arrest protesters, especially during the 2018 city riots.
Another controversial case concerns the surveillance equipment used on the US-Mexico border, such as the towers in Arizona that allow to monitor people up to 12km away.
The images captured by these installations are analyzed with artificial intelligence and it is not so clear whether their use is legally legitimate.
Will the Western world be able to strike a balance between these issues?
I’m gonna ask my Palantir!